The Laboratory for Intelligent Systems and Structural Analysis (ISSA Lab) was founded at HSE University in 2012 by Prof. Sergei O. Kuznetsov.
ISSA Lab is a part of the School of Data Analysis and Artificial Intelligent at the Faculty of Computer Science. Laboratory staff participates in all academic activities of the Faculty including bachelor's and master's programmes, as well as the Doctoral School of Computer Science (thesis preparation).
The Laboratory conducts fundamental and applied research in data analysis of large volume and complex structure of data; software tools for data analysis and components of intelligent systems are being developed.
The Laboratory is developing into a centre for research and integration of the latest artificial intelligence methods (with an emphasis on data mining and structural analysis) to increase "intelligence" and achieve adaptability of software tools.
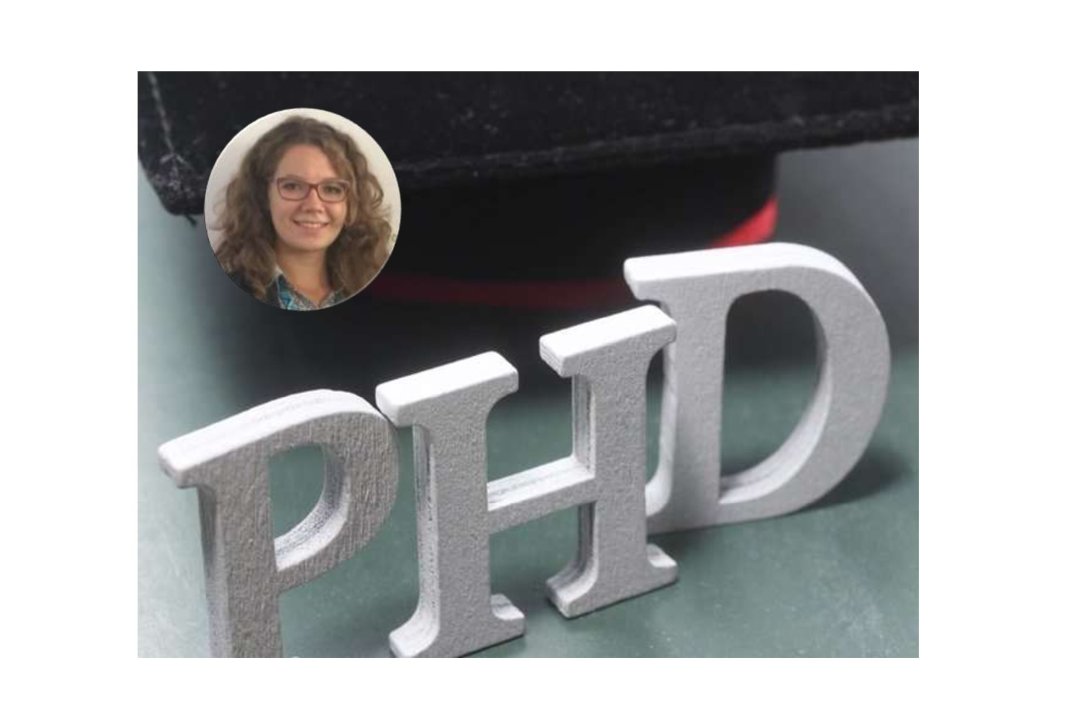
Lisa Goncharova successfully defended her dissertation
On September 26, 2022, Elizaveta Goncharova defended her PhD thesis.
.jpg)
International seminar "HAO Intelligence for Big Wisdom"
An international seminar was held on May 6, 2022.