IEEE BIBM 2020 conference
Fedor Pavlov, a research intern at the International Laboratory of Bioinformatics, gave a presentation at the IEEE BIBM 2020 international conference, which was held online December 16-19, 2020. At the Machine Learning and Artificial Intelligence in Bioinformatics and Medical Informatics (MABM) workshop, he presented a paper "Recognition of DNA Secondary Structures as Nucleosome Barriers with Deep Learning Methods," published in co-authorship with Maria Poptsova.
In their work, the scientists marked up DNA sites where G-quadruplexes can act as nucleosome barriers, compiled a task to classify such sites using deep learning methods based on convolutional and recurrent neural network architectures, and interpreted the convolutional layer filters of the neural network to identify motifs that may indicate the presence of nucleosome barriers.
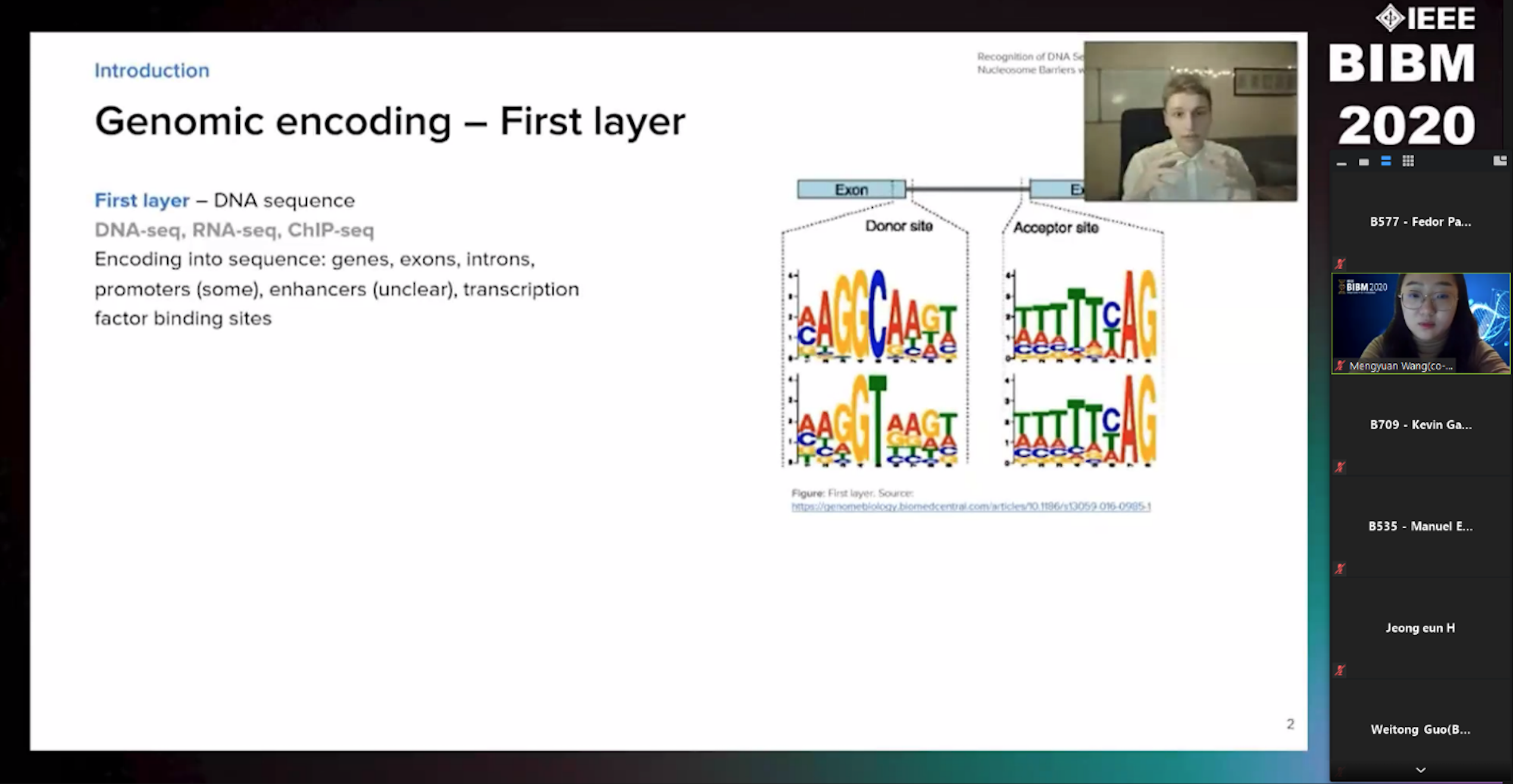
Over the past few years, genome research using machine and deep learning techniques has become increasingly popular. Recognition of patterns of DNA secondary structures and genomic functional elements are still poorly investigated, even though research in this area has the potential to contribute greatly to the development of medicine and pharmacology.
DNA secondary structures may affect various genomic processes such as transcription, translation, and replication. One of the mechanisms of transcriptional regulation is the regulation of nucleosome positioning. Some DNA structures can compete with nucleosomes for location in the genome and even serve as barriers separating nucleosomes. Nowadays, both the problem of nucleosome positioning and the problem of DNA secondary structures detection is trying to be solved using both machine and deep learning methods.
This study aimed to explore machine and deep learning methods that have proven to be successful in natural language processing for the task of DNA sequence recognition. In this study, two deep learning models based on the results of the testing were selected based on CNN architectures and a combination of convolutional and recursive CNN and LSTM architectures, respectively.
To test the models, four binary and multiclass classification problems were composed. In each case, the input data was a set of nucleotide sequence segments of fixed length. Within each problem, the segments were classified into predetermined classes. The main classes for the study were divided into segments in which nucleosomes, G-quadruplexes, and patterns of secondary structures relative to nucleosomes were present. The best classification results according to the test results were achieved on the model with CNN and LSTM architecture.
At the final stage of the study, the filters of the input convolutional layer of the neural network were interpreted. The data from each filter of the trained deep learning model was used for the analysis. The interpretation resulted in a set of 16 motifs, which were subsequently tested for matches with already studied motifs.