NeurIPS — 2020 Accepts Three Articles from Faculty of Computer Science’s Researchers
34th conference on neural information processing systems NeurIPS 2020 is one of the largest conferences on machine learning in the world, taking place since 1989. It was going to take place in Vancouver, Canada on December 6-12, but takes place online.
NeurIPS is as prestigious as ever, with 9,454 articles submitted and 1,300 articles accepted by the conference. Among those accepted are three articles by the researchers of the Faculty of Computer Science:
Black-Box Optimization with Local Generative Surrogates by LAMBDA researchers Vladislav Belavin and Andrey Ustyuzhanin.
On Power Laws in Deep Ensembles by Samsung-HSE Lab researchers Ekaterina Lobacheva, Nadezhda Chirkova, Maxim Kodryan and Dmitry Vetrov.
Towards Crowdsourced Training of Large Neural Networks using Decentralized Mixture-of-Experts by Yandex Lab researcher Maxim Ryabinin.
We asked the authors to tell us about their researches.
Nadezhda Chirkova
Research Fellow
In our research, we investigate the laws that determine the changes in the quality of the ensembles of deep neural networks. Ensembling is a simple procedure that consists of the training of multiple neural networks and averaging the results. Averaging helps to reduce the number of errors of the model as well as to evaluate more precisely whether the model is certain of its predictions or it is better to use an alternative source of information. For example, a smartphone’s fingerprint recognition model can be successful 99% of the time and refuse to make a prediction 1% of the time, asking for the password instead. The more neural networks we ensemble, the higher the quality of the prediction is. The law we have discovered allows to determine the minimal number of neural networks necessary for the required quality of predictions. In our research, we actively used computation resources of HSE University’s Supercomputer Simulation Unit.
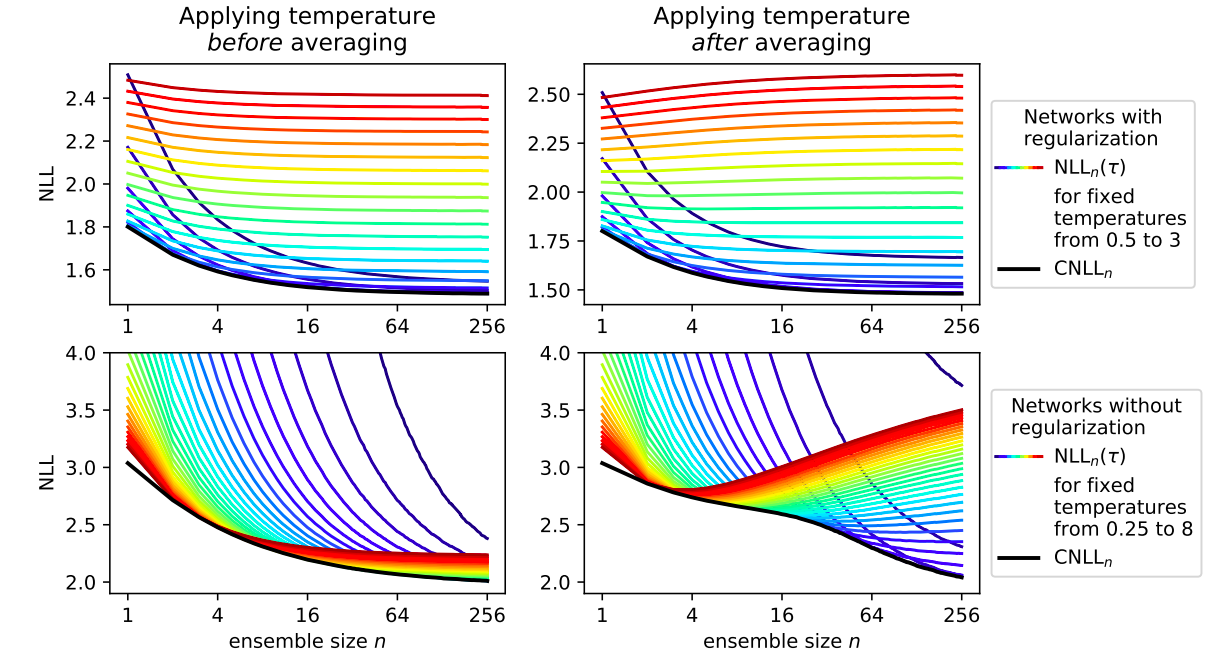
Andrey Ustyuzhanin
Laboratory Head
Many cases in contemporary physics and engineering can be simulated by non-differentiable simulators with intractable likelihoods. Optimisation of such models in parameter space P is very challenging, especially when the simulated process is stochastic. To solve this problem, we introduce deep generative surrogate models to iteratively approximate the simulator in local neighbourhoods of the parameter space P.
It is a more than year-long journey mainly driven by Sergey Shirokobokov (Imperial College London), Michael Kagan (SLAC National Accelerator Laboratory) and Atilim Gunesh Baydin (University of Oxford). It has started as a discussion at ACAT 2019 conference at Saas-Fee, Switzerland.
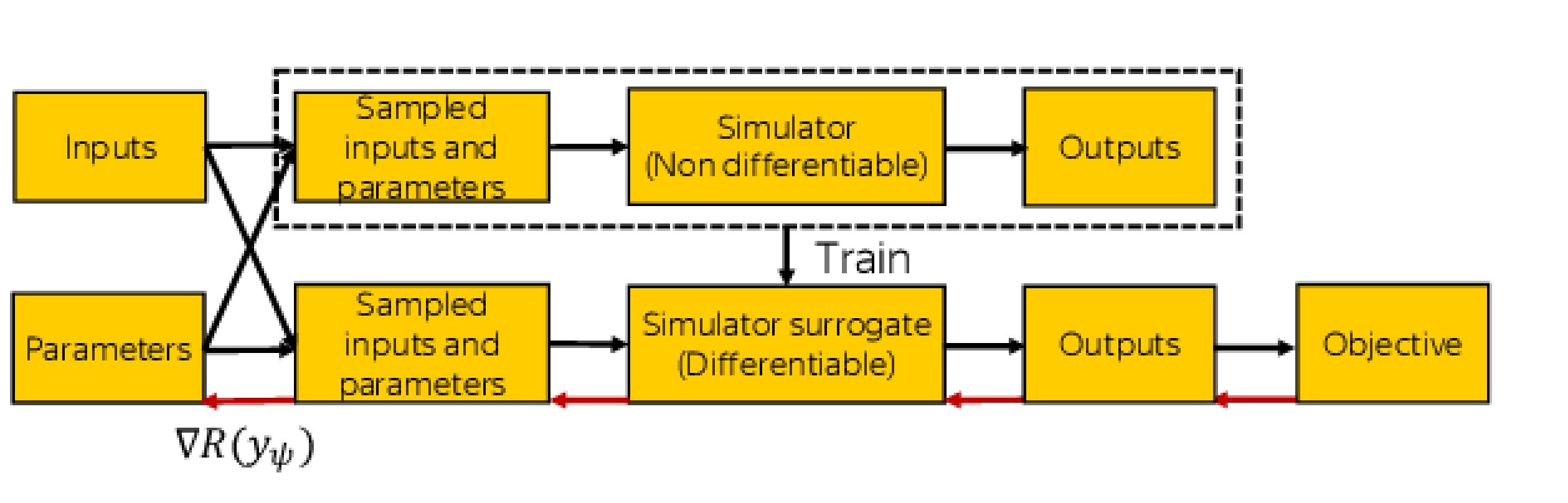
Maxim Ryabinin
Research intern, Yandex Laboratory
There is a real arms race in deep learning in the last couple of years: giant corporations (Google, Microsoft, NVIDIA, OpenAI) break records of the size of trained language models every month. This leads to ever greater results. Because of the computational costs, training of these models can cost millions of dollars, while the models contain so many parameters that it is challenging even to place them in the users’ GPU. Suchlike trend leads to the non-reproducibility of new deep learning results and their inaccessibility for the independent researchers and institutions with lesser computational power. Other disciplines have learned to solve this problem: projects like Folding@home allow the researchers to use the free computational resources of the volunteers’ computers to solve important and complex tasks.
Our purpose was to propose a similar approach for machine learning taking into account the specific tasks and data exchange necessary for the solution. The proposed approach allows neural network training on participants’ computers connected via the Internet even if the parameters of the problem cannot be placed in any single device. Specialised Decentralized Mixture-of-Experts layer that we developed for this purpose allows for stable learning in case of network delays and connection disruption. Particularly, we adapted distributed hash tables to ensure the stability of distributed learning – a technique popularised by BitTorrent file exchange protocol.
We have submitted our article in June and received the first reviews in early August. When we gave our replies to the reviewers they reevaluated our research higher – that’s probably why our article was accepted in the end.
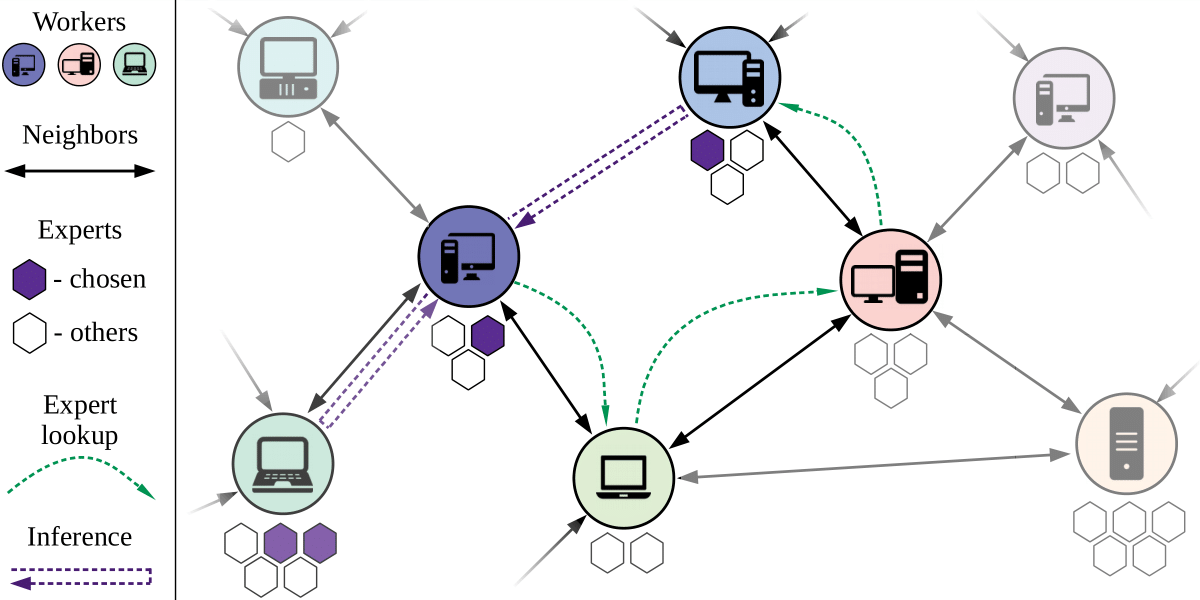
Vladislav Belavin
Ekaterina Lobacheva
Andrey Ustyuzhanin
Nadezhda Chirkova